The Importance of Training Data for Self-Driving Cars
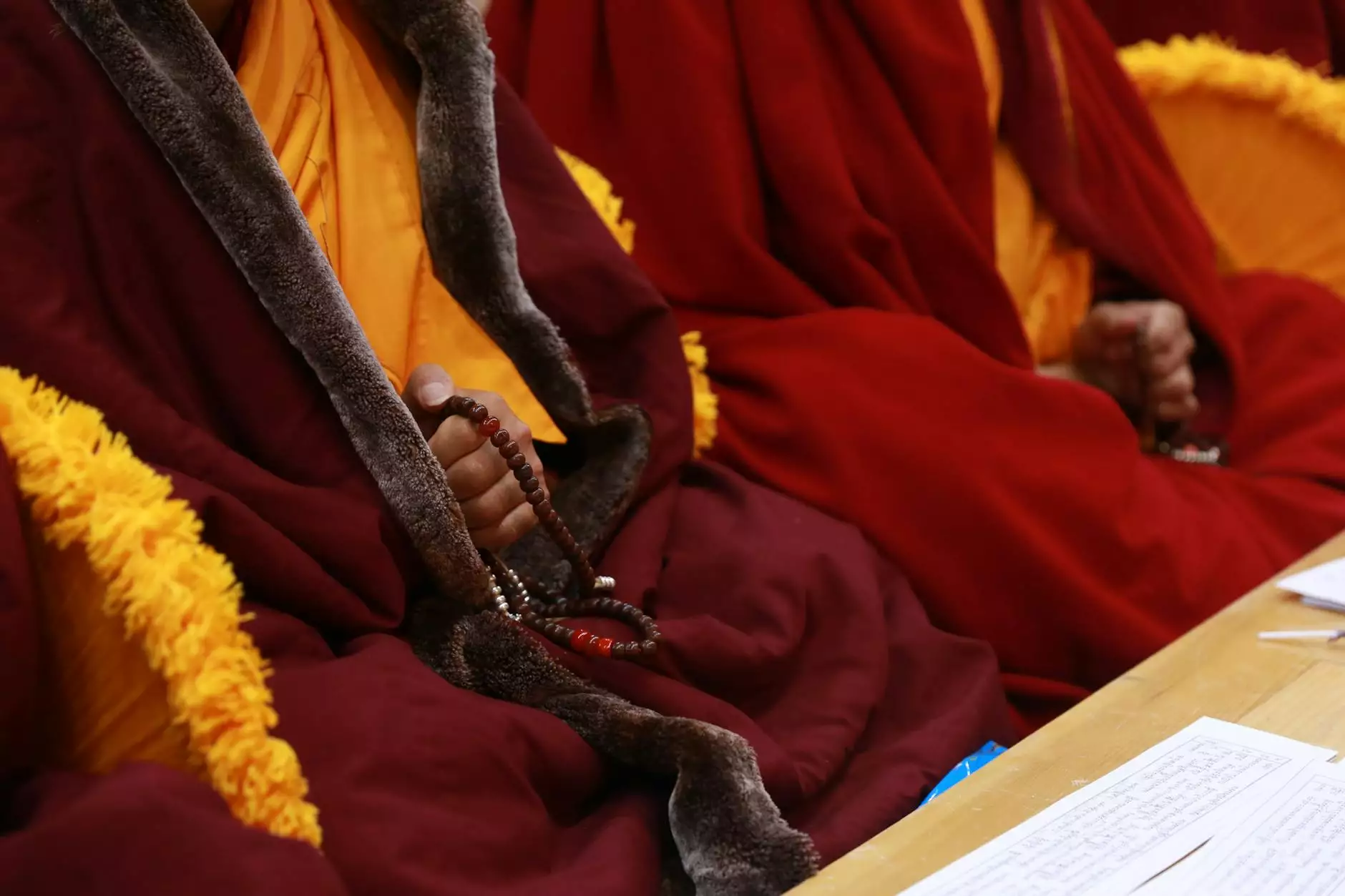
Self-driving cars are not just a futuristic concept but a rapidly advancing technology that promises to reshape the automotive landscape. At the core of these advancements lies the concept of training data for self-driving cars. The intricacies of this data and its applications are crucial for understanding how autonomous vehicles operate safely and efficiently.
What is Training Data?
Training data is a set of data used to train algorithms and machine learning models. In the context of self-driving cars, this data teaches the vehicles how to interpret their surroundings, make decisions, and navigate various driving conditions.
The Role of AI and Machine Learning in Autonomous Vehicles
Artificial Intelligence (AI) and machine learning play pivotal roles in the operation of self-driving cars. These technologies rely heavily on vast amounts of training data to achieve higher levels of accuracy and reliability.
Types of Training Data
- Visual Data: Images and videos from various cameras placed on the vehicle.
- Sensor Data: Information from Lidar, radar, and ultrasonic sensors that help in comprehending the environment.
- Geospatial Data: Mapping data that provides context about locations, road layouts, and traffic patterns.
- Behavioral Data: Information about how other drivers behave in different situations, which is crucial for predicting actions on the road.
How Training Data is Collected
The collection of training data for self-driving cars involves multiple methods:
1. Real-World Driving
Test vehicles equipped with numerous sensors and cameras drive around various environments, gathering data on everything from road conditions to the behavior of other road users.
2. Simulation Environments
Advanced simulations replicate different driving scenarios, allowing developers to test their algorithms without the risks associated with real-world driving.
3. Public Data Sets
Researchers and companies may use publicly available data sets to enrich their training data, allowing for a broader understanding of driving dynamics.
Challenges in Training Data Acquisition
While collecting training data is essential, it also presents several challenges:
- Volume: The sheer amount of data required for effective training can be overwhelming.
- Diversity: The data must cover various scenarios, weather conditions, and geographical locations to ensure robustness.
- Quality: High-quality data is necessary; poor data can lead to ineffective or dangerous driving decisions.
- Data Privacy: Collecting data in public spaces raises questions about privacy and consent.
Significance of Quality Training Data
The quality of training data directly influences the performance of self-driving cars. Poorly trained models can lead to:
- Inaccurate Decision Making: A lack of diverse data can cause vehicles to misinterpret scenarios, leading to unsafe outcomes.
- Increased Accident Risks: If an autonomous vehicle cannot predict the behaviors of other drivers accurately, it may result in collisions.
- Limited Functionality: Without comprehensive training, vehicles may be unable to operate effectively in various weather conditions or complex urban environments.
The Future of Training Data for Self-Driving Cars
As technology evolves, so does the training data for self-driving cars. The future will likely see:
- More Advanced Sensor Technologies: New types of sensors will enhance the quality of data collected.
- Collaboration with Smart Cities: Cities may provide data to vehicles to improve navigation and efficiency.
- Continuous Learning: Self-driving cars will continuously learn and update their models based on new data collected during everyday operation.
The Impact of Training Data on Road Safety
One of the primary goals of developing self-driving cars is to improve road safety. Properly trained autonomous vehicles can:
- Reduce Human Error: The majority of road accidents are caused by human error; enhancing training data could significantly reduce these incidents.
- Enhance Responsiveness: AI can process information faster than a human, allowing vehicles to react more quickly to potential hazards.
- Improve Traffic Management: Self-driving cars can communicate with each other to optimize traffic flow and reduce congestion.
Real-World Applications of Self-Driving Cars
Self-driving cars have already begun to make their presence felt in various sectors:
1. Ridesharing
Companies like Waymo and Uber are investing heavily in self-driving technology, looking to revolutionize the ridesharing experience.
2. Delivery Services
Autonomous vehicles are being tested for delivering goods, enhancing logistics efficiency.
3. Public Transportation
Self-driving buses and shuttles are being introduced in several cities to provide convenient public transport options.
Conclusion
The journey of training data for self-driving cars is ongoing and filled with potential. As more data is collected, algorithms will become even more sophisticated, paving the way for a safer and more efficient future in transportation. The advancement of this technology will not only change how we think about driving but will also reshape entire industries.
To stay ahead in the evolving landscape of self-driving technology, businesses in fields like Home Services, Keys & Locksmiths, must also consider the implications of this transformation. Adapting to these changes will be crucial for maintaining relevance in a rapidly approaching autonomous future.
training data for self driving cars