Harnessing the Power of Data Labeling in Machine Learning
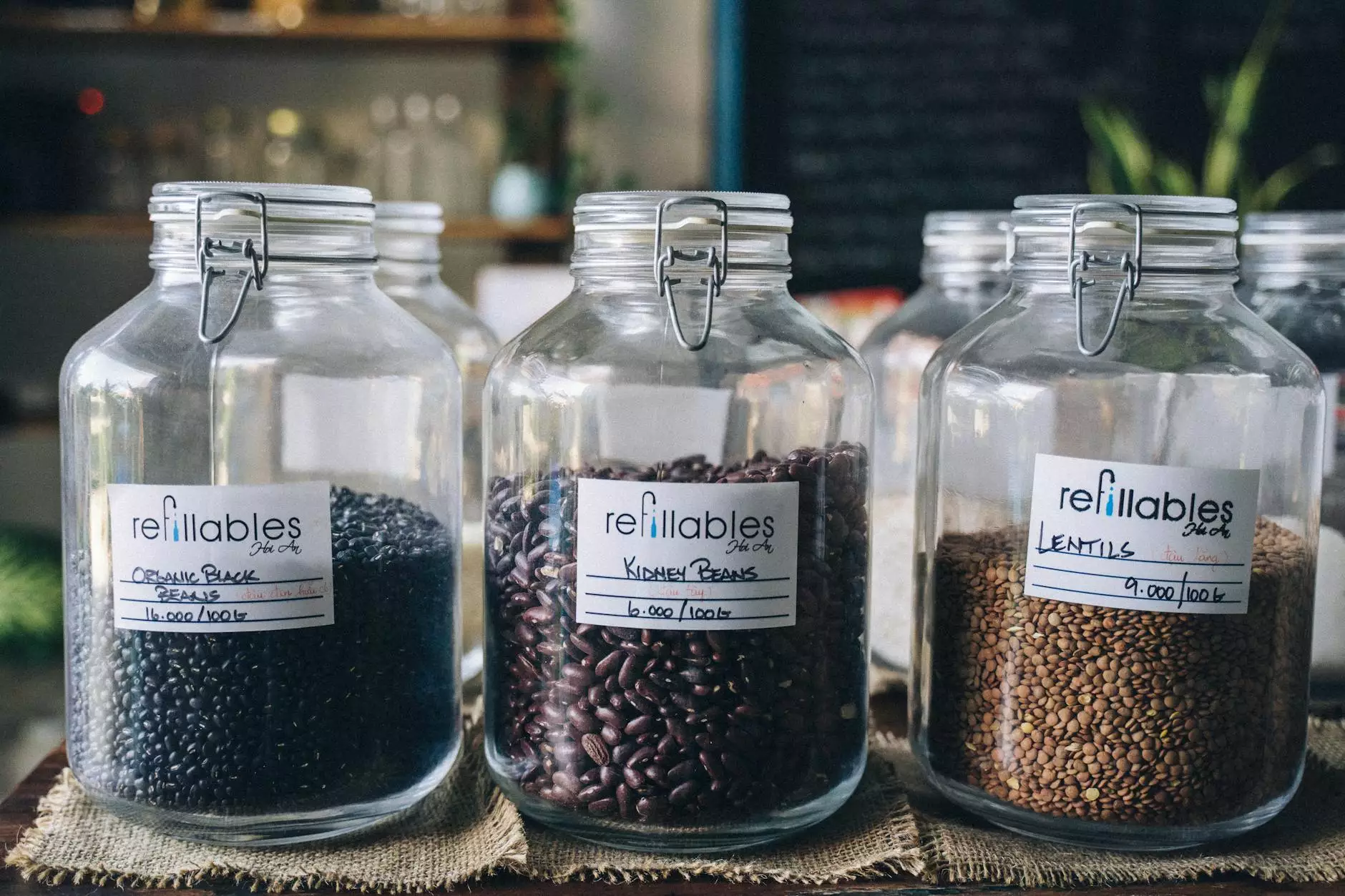
In the digital age, where data is hailed as the new oil, the importance of data labeling machine learningcannot be overstated. This process is crucial for training sophisticated algorithms and ensuring that artificial intelligence (AI) operates effectively. As businesses and organizations turn to AI to drive innovation, understanding the critical role of data labeling is more important than ever.
Understanding Data Labeling
Data labeling refers to the process of annotating data—whether it’s text, images, videos, or audio—to provide context that machine learning models can interpret. By tagging data with relevant labels, AI systems can learn to recognize patterns and make decisions based on the labeled data. This operation is fundamental for various AI applications, including natural language processing, computer vision, and speech recognition.
The Importance of Data Labeling in Machine Learning
Accurate data labeling machine learning directly influences the performance of AI models. Here are some key reasons why data labeling is essential:
- Improved Accuracy: Well-labeled data enhances the precision of machine learning models, leading to better decision-making processes.
- Efficiency in Training: Data labeling streamlines the training of AI algorithms, allowing them to learn from clear and organized datasets.
- Facilitates Supervised Learning: Many machine learning models require labeled data to improve over time, making data labeling a necessity.
- Quality Data Equals Quality Outcomes: The effectiveness of an AI model significantly correlates with the quality of the data it was trained on.
Types of Data Labeling Techniques
Data labeling can employ various techniques depending on the type of data involved:
1. Image Annotation
This involves identifying and labeling objects within images. Common techniques include bounding boxes, polygons, and landmarking.
2. Text Annotation
Text data can be labeled for various purposes, such as sentiment analysis, where labels like positive, negative, or neutral are applied based on the context of the content.
3. Audio Annotation
Involves labeling segments of audio data for tasks such as speech recognition or music classification. This might include identifying speakers or marking specific sounds.
4. Video Annotation
This refers to the process of labeling video segments, often done in object tracking applications, where objects in the video are identified and labeled frame-by-frame.
Challenges in Data Labeling
Despite its significance, data labeling comes with its set of challenges:
- Time-Consuming: Manual data labeling is labor-intensive and can be a bottleneck in the data preparation process.
- Consistency Issues: Different annotators may label the same data differently, leading to inconsistencies that could affect model training.
- Scalability Problems: As datasets grow, scaling the labeling process becomes increasingly difficult without the right tools.
Overcoming Data Labeling Challenges with Technology
To address the challenges associated with data labeling, many companies are turning to advanced tools and platforms. Keylabs.ai offers an innovative solution by providing a robust data annotation tool that streamlines the labeling process.
Key Features of Keylabs.ai's Data Annotation Platform
- Automated Labeling: By leveraging machine learning algorithms, Keylabs.ai can automate parts of the labeling process, significantly reducing time and effort.
- Collaboration Tools: The platform enables collaboration among teams, ensuring consistent labeling across projects.
- Scalable Solutions: Keylabs.ai can handle large datasets, making it easier for businesses to scale their machine learning operations.
- Real-time Insights: Businesses can track the status of their data labeling projects in real-time, ensuring transparency and efficiency.
Applications of Data Labeling in Various Industries
The applications of data labeling machine learning extend across various sectors:
1. Healthcare
In the medical field, data labeling is used to annotate medical images for disease detection, enabling AI models to assist radiologists in diagnosing conditions more accurately.
2. Automotive
Self-driving cars rely heavily on meticulously labeled data to understand and navigate the environment around them, enhancing safety and reliability.
3. Finance
Financial institutions use data labeling to detect fraudulent transactions and assess credit risks, facilitating safer financial environments.
4. Retail
Retailers leverage data labeling in customer behavior analysis and inventory management, optimizing supply chain processes.
Best Practices for Effective Data Labeling
To maximize the effectiveness of data labeling efforts, consider implementing the following best practices:
- Define Clear Guidelines: Establish comprehensive labeling guidelines to ensure uniformity and consistency among annotators.
- Invest in Training: Properly train your labeling team to familiarize them with standards and practices.
- Utilize Advanced Tools: Leverage advanced data annotation platforms like Keylabs.ai to enhance labeling efficiency and accuracy.
- Continuous Monitoring: Regularly review labeled data to ensure high quality and address any inconsistencies that may arise.
The Future of Data Labeling in Machine Learning
As the demand for quality labeled data grows, the future of data labeling is set for transformation. Automation, driven by AI and machine learning technologies, is paving the way for quicker and more efficient labeling processes. Furthermore, advancements in natural language processing and computer vision are expected to enhance the capabilities of data labeling tools, making them more intuitive and user-friendly.
Conclusion
In summary, data labeling machine learning is a vital component in harnessing the full potential of artificial intelligence. By improving model accuracy and efficiency, businesses can achieve better outcomes across various applications. Organizations like Keylabs.ai are leading the way with innovative data annotation tools and platforms that promise to simplify and enhance the labeling process. Embracing these technologies not only addresses current challenges but also prepares businesses for future advancements in AI technology.
As data continues to expand exponentially, the need for effective and efficient data labeling solutions will only continue to grow. Investing in quality labeling today is preparing for a smarter, more data-driven future.