Unlocking Insights: The Role of Agriculture Datasets in Machine Learning
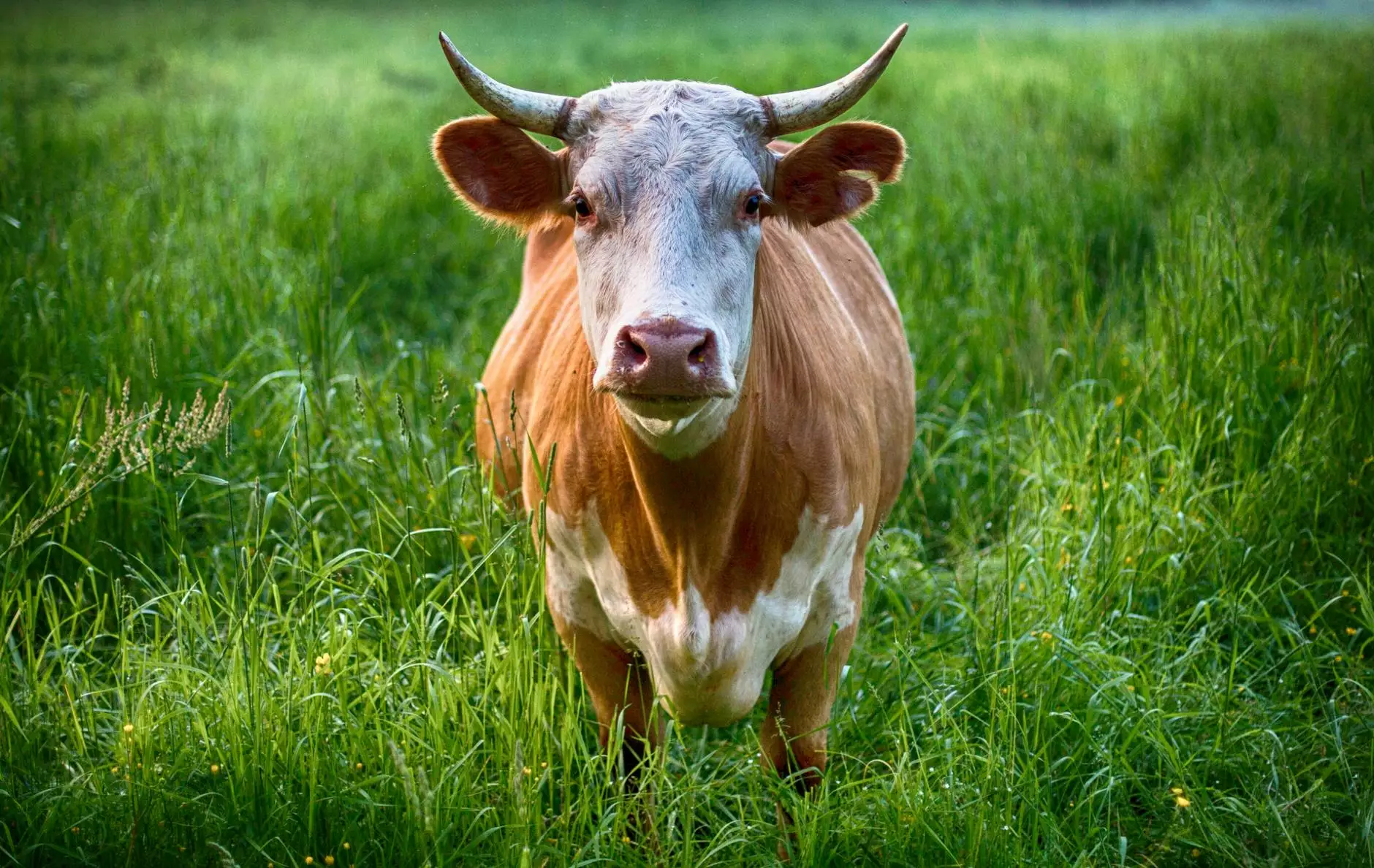
With the rapid advancements in technology and data science, agriculture datasets for machine learning are becoming indispensable tools for enhancing productivity and innovation in the agricultural sector. This article delves deep into how these datasets are transforming agriculture, the potential they hold for businesses, particularly in the fields related to Home Services, Keys & Locksmiths, and how organizations can leverage them to make informed decisions.
Understanding Agriculture Datasets
Agriculture datasets comprise an array of information that pertains to various aspects of the agricultural industry, including:
- Crop Yields: Historical yield data for various crops.
- Soil Conditions: Information about soil pH, moisture levels, and nutrient content.
- Weather Patterns: Data on precipitation, temperature, and climate conditions.
- Pest and Disease Incidence: Records of outbreaks affecting crop health.
- Market Trends: Prices and demand fluctuations for agricultural products.
The Importance of Agriculture Datasets in Machine Learning
Machine learning (ML) utilizes algorithms to analyze vast amounts of data and predict future outcomes based on historical trends. In agriculture, datasets serve as the foundation upon which predictive models are built. This approach allows stakeholders to:
- Optimize Crop Production: By analyzing historical yield data alongside weather and soil conditions, farmers can make data-driven decisions about when and how to plant crops.
- Enhance Resource Management: Machine learning models can predict irrigation needs, optimizing water usage and reducing costs.
- Identify Pest Threats Early: Data on pest occurrences can be used to build early warning systems, allowing producers to take proactive measures.
Potential Applications of Agriculture Datasets
The applications of agriculture datasets in machine learning span multiple domains. Here are some notable examples:
1. Precision Agriculture
Precision agriculture includes an array of technologies and practices aimed at increasing agricultural productivity. By employing agriculture datasets for machine learning, farmers can create detailed maps that highlight variations in crop performance across fields. Techniques like remote sensing, coupled with data analytics, enable:
- Targeted Interventions: Farmers can apply fertilizers and pesticides only where needed, minimizing waste and environmental impact.
- Yield Prediction: Predictive analytics can offer insights into expected yields based on crop types, soil health, and historical weather patterns.
2. Supply Chain Optimization
Agricultural businesses, suppliers, and retailers can utilize datasets to enhance their supply chains. Machine learning algorithms can analyze market trends, demand patterns, and logistics to help businesses:
- Forecast Demand: Accurate demand forecasting enables better inventory management, reducing losses due to spoilage.
- Optimize Delivery Routes: Machine learning can identify the most efficient delivery routes, saving time and fuel costs.
3. Climate Resilience
With climate change significantly affecting agricultural practices, datasets developed through machine learning can play a pivotal role in building resilience. By understanding historical climate patterns and predicting future conditions, farmers can:
- Adopt Sustainable Practices: Implement practices suited to changing climatic conditions.
- Plan Crop Rotations: Choose the right crops for the right seasons, maximizing productivity while minimizing environmental impact.
Benefits for Businesses Related to Home Services, Keys & Locksmiths
For businesses in the Home Services, Keys & Locksmiths sectors, the insights gained through agriculture datasets for machine learning may seem indirect but are immensely valuable:
Enhanced Security Solutions
Understanding local agricultural patterns can help locksmith companies provide improved security solutions tailored to local needs. For instance:
- Itinerant Workers: Anticipating the influx of seasonal workers can prompt locksmiths to offer tailored security services, enhancing the overall safety of residences and properties.
- Remote Management: Businesses can leverage IoT devices integrated with machine learning algorithms to offer smarter locking solutions, catered to changing agricultural needs.
Data-Driven Marketing Strategies
Using insights from agricultural datasets, locksmith businesses can create more targeted marketing strategies. Understanding the seasonal demands of farmers and home services can enable:
- Customized Promotions: Offering discounts or services that align with agricultural cycles, such as securing barns and equipment during harvest season.
- Localized Advertising: Tailoring advertising efforts based on regional agricultural activities helps businesses reach potential clients more effectively.
Challenges in Utilizing Agriculture Datasets
While the benefits are substantial, the utilization of agriculture datasets for machine learning does come with challenges:
Data Accessibility and Quality
One of the significant hurdles is ensuring the availability of high-quality data. Many datasets may be incomplete, outdated, or not readily accessible, which can hinder effective analysis. Addressing these challenges involves:
- Investing in Data Collection: Encouraging the development of comprehensive datasets through collaboration among government agencies, research institutions, and private entities.
- Data Standards: Establishing clear standards for data collection and sharing can enhance the overall quality of datasets in agriculture.
Ethical Considerations
The use of agriculture datasets also brings forth ethical considerations regarding data privacy and security. Stakeholders must navigate:
- Data Ownership: Defining who owns the data and how it can be used, especially when dealing with sensitive agricultural data.
- Transparency and Trust: Building trust among farmers and businesses is crucial for successful data sharing and collaboration.
Conclusion: Embracing the Future of Agriculture with Data
In conclusion, leveraging agriculture datasets for machine learning presents immense potentials for businesses in agriculture and related sectors, like Home Services, Keys & Locksmiths. As we advance toward a future where data-driven decisions become imperative, the ability to harness these datasets will distinguish businesses able to capitalize on opportunities from those that fall behind. By understanding the intricacies of datasets and implementing innovative technologies, businesses can unlock new levels of efficiency, sustainability, and profitability in the ever-evolving agricultural landscape.
Embracing the challenges, optimizing resource management, and blending technology with traditional practices can lead to a more sustainable and prosperous agricultural future. The integration of machine learning into agriculture is not merely a technological advancement; it signals a transformation that has the potential to revolutionize the agricultural landscape for generations to come.
agriculture dataset for machine learning